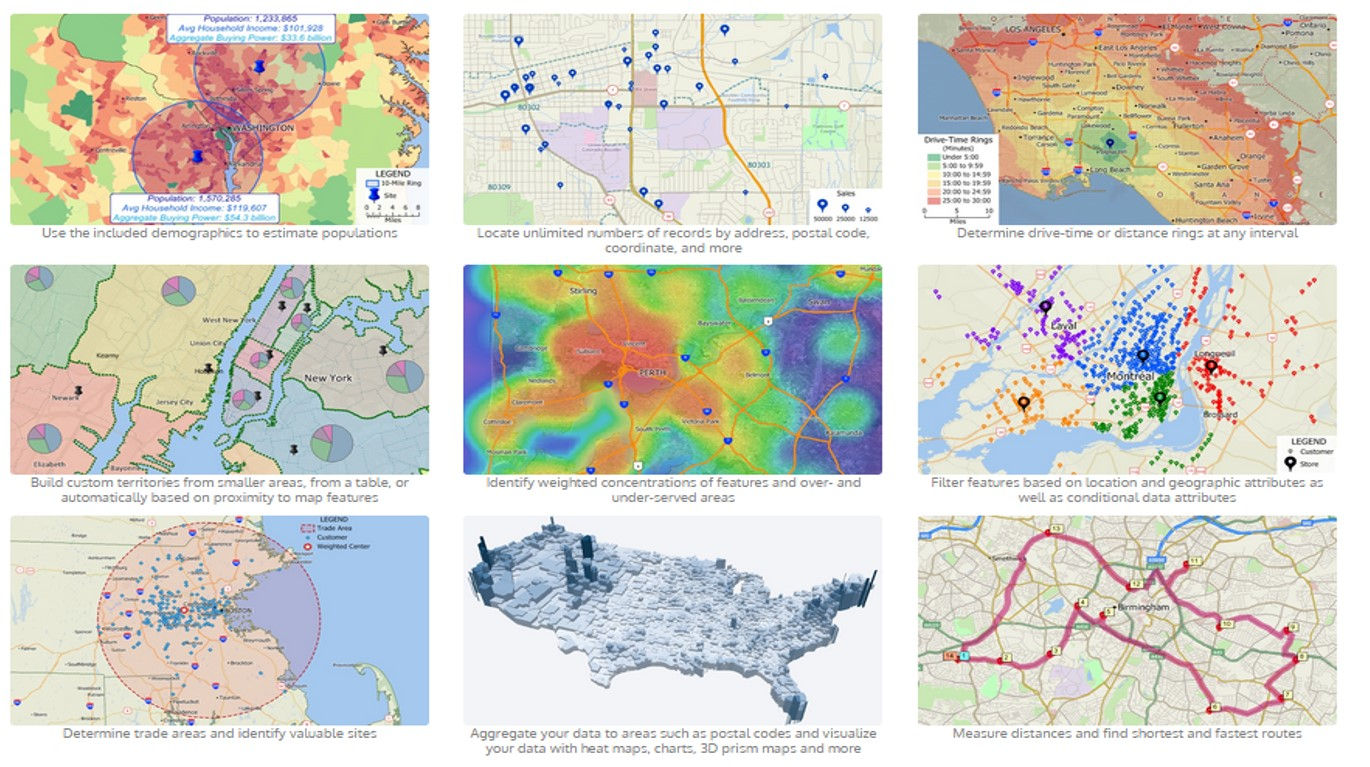
To research the classification based on the satellite image in ERDAS IMAGINE, I used a satellite image Landsat Surface Reflectance – L8 OLI/TIRS which I downloaded from the site http://landsat.usgs.gov/. Date of my image July 16, 2015, summer, because at that time a lot of vegetation. I chose the area of district Talas, Kyrgyz Republic. I tried to choose the area where there is a forest, river, pond, pasture, settlements and agricultural areas
Classification in ERDAS IMAGINE program
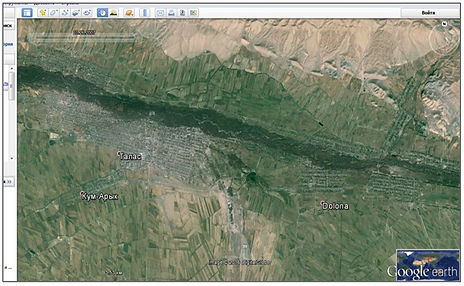
Classification is the process of sorting pixels into a finite number of individual classes, or categories, of data based on their data file values. If a pixel satisfies a certain set of criteria, then the pixel is assigned to the class that corresponds to that criteria. There are two ways to classify pixels into different categories:
-
Supervised;
-
Unsupervised.
Unsupervised is more computer-automated. It enables you to specify some parameters that the computer uses to uncover statistical patterns that are inherent in the data. These patterns do not necessarily correspond to directly meaningful characteristics of the scene, such as contiguous, easily recognized areas of a particular soil type or land use. They are simply clusters of pixels with similar spectral characteristics. In some cases, it may be more important to identify groups of pixels with similar spectral characteristics than it is to sort pixels into recognizable categories.

Supervised classification
Supervised classification is more closely controlled by you than unsupervised classification. In this process, you select pixels that represent patterns you recognize or can identify with help from other sources.
We must select pixels that are recognized as representative of the classes set out in the classification scheme. Thus, training area identified in the field or on aerial photographs or maps have corresponding training pixels in the feature set image. Training helps the supervised classification find similar patterns (i.e., signatures) throughout the remainder of the image. Parametric signatures are composed of statistical descriptions of the spectral reflectance properties of a class (mean and covariance matrix) based on the pixels in the training sample (the collection of all training pixels for an individual class). Parametric signatures are used to train a statistically based classified/decision rule (e.g., Maximum Likelihood classifier). Every pixel is assigned to a class using these parametric rules. I have an empty signature editor in which I will create and edit signatures.
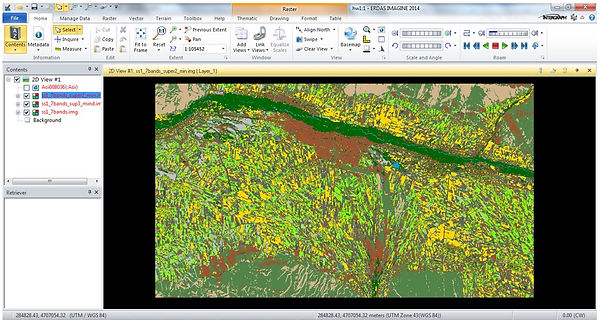
Conclusion
The primary objective of this research was make supervised classification and unsupervised classification using different methods in Erdas. I chose more familiar training area, because I think the most important factor in deciding which classification to use is knowledge about training area. My classes for classification were urban area, pasture, agricultural area, forest, water and clay area without vegetation. For supervise classification I used three methods Minimum Distance, Maximum Likelihood and Mahalanobis Distance. I think Minimum Distance method give to me good result. In the case Maximum Likelihood and Mahalanobis Distance water class was dispersed, also forests, agriculture with dense vegetation decreased. Agriculture area with less vegetation covered the area of water, forests, clay surface. Result of Minimum Distance more like a reality. I also used Google Earth in case of difficulties with the classification definition.
I think if operator is not intimately familiar with training area, unsupervised classification would most likely be more effective.